ACCURACY AND EQUITY IN PREDICTIVE HOTSPOT POLICING
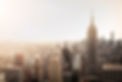
MOTIVATION
Several recent studies have demonstrated the efficacy of proactive policing strategies for crime prevention. By predicting emerging geographic hot-spots of violent crime, we can target police patrols and other interventions. However, predictive policing creates moral and ethical concerns, such as fairness and equity, which have been well-documented, yet have not been typically incorporated into the design and evaluation of such systems. In this work we develop machine learning methods to predict hot-spots of crime and present a way to measure equity among those areas. We then adjust the predictions based on the defined equity metric and analyze the trade-offs between accuracy and equity. We also see the performance of the two models based on the racial distribution of the population.

KEY QUESTIONS

RESEARCH APPROACH
Predictive Modeling
We employed three machine learning modeling techniques for crime prediction. Through our prediction model we aim at forecasting the weekly crime numbers of Part 1 violent crimes in a city for a given census tract. Part 1 crimes include murder and non-negligent homicide, rape, robbery, aggravated assault, burglary, motor vehicle theft, larceny-theft, and arson
LSTM MODEL
Modeled using the aggregated crime numbers of NYC based on last 10 years
Novel approach of applying time-series clustering for census-tracts, and applying separate models for each of those clusters.
Three model architectures with best performing model having 100 units, two output Dense layers with 'ReLU' and Sigmoid non-linearities along with dropout.
GAUSSIAN PROCESS MODEL
Models spatial dependencies using data from neighboring census tracts as features
Also uses eight years of temporal information as features.
Model optimizes for Radial Basis Function (RBF) kernel with average length of 5 time steps, the Exponential Kernel with average periodicity of 52, and the White Noise kernel with length 0.1.
RANDOM FOREST MODEL
Geographical information like existence of Parks or vacant plots/buildings, Police stations, Transportation/Health facilities as features
Eight years of historical data
High performance
Equity Metric
Equity in our analysis is based on the premise that all members of the community, regardless of their race, ethnicity or economic status receives the same policing services. We have defined equity in terms of policing metric that balances the demand and supply of policing in a neighborhood.

RESULTS
CONCLUSION
Through our work, we predicted weekly numbers of Part 1 violent crimes at a census tract level. We explored three different methodologies for crime prediction: An LSTM model incorporating the seasonality of crimes, a gaussian process model taking into account spatial correlation and a random forest model which uses the geographic features of the neighborhood as predictors. Our results show that the random forest model performs better in capturing more crimes by intervening specific tracts.

POLICY IMPLICATION
Our an extension of our weekly predictions can be used to assess short term risk and aid in deployment of patrolling. Our model specifically would tell top census tracts to intervene in a given week. The number of such census tracts to be intervened can be decided based on the available resources. The efficacy of these results would depend on choice of intervention and ground deployment.
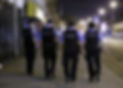
LIMITATIONS
Even though we haven’t directly used any indicators of protected groups(Like race/ethnicity, gender),associations with historical crime numbers can bring in bias. The model has achieved better predictive accuracy, but the underlying causal effect cannot be deduced from the analysis. So the work only gives empirical evidence that incorporating geographical features gives higher accuracy.
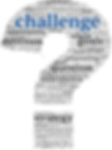
About US
ANUPAMA SANTHOSH
Masters's Student, NYU CUSP
DEVASHISH KHULBE
Master's Student, NYU CUSP
YAVUZ SUNOR
Master's Student, NYU CUSP
YUCHEN DING
Master's Student, NYU CUSP